
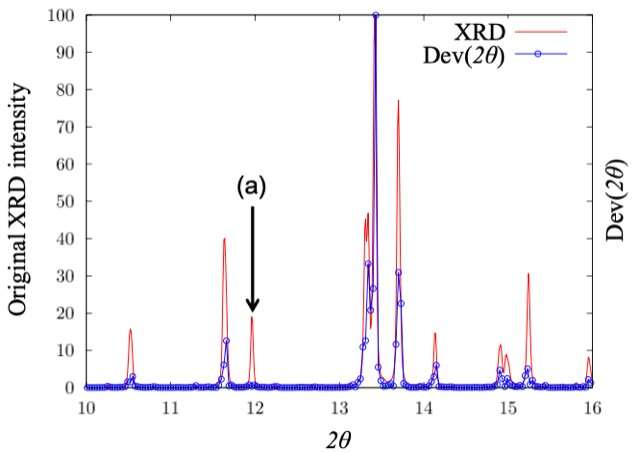
X-ray diffraction (XRD) is an experimental method to discern the atomic construction of a cloth by irradiating it with X-rays at totally different angles. Basically, the depth of the mirrored X-rays turns into excessive at particular irradiation angles, producing a sample of diffraction peaks. An XRD serves as a fingerprint for a cloth since every substance produces a singular sample.
In analysis and growth, modifications in XRDs are used to determine the positions and quantities of further components that have to be added to fine-tune a cloth to assist improve a desired practical property, say, vitality storage effectivity in batteries.
Nevertheless, the height modifications in XRDs are barely discernible to people. This makes ascertaining the options and relevance of various peaks for materials characterization troublesome. To this finish, a gaggle of Japanese researchers, led by Professor Ryo Maezono from the Japan Superior Institute of Science and Expertise (JAIST), utilized a Deep Studying method referred to as “auto-encoder” to the issue to seek out hidden regularities in XRDs that would assist speed up the event of recent practical supplies.
The analysis group additionally included Affiliate Professor Kenta Hongo and Assistant Professor Kousuke Nakano from JAIST. Their work has been printed in Superior Concept and Simulations.
Explaining the basics of the auto-encoder method, Prof. Maezono says, “The auto-encoder method captures knowledge options by expressing them as factors on a two-dimensional aircraft (function area). Based mostly on their scatter, the factors get grouped to coarse-grain info. The auto-encoder compresses the information dimension and may effectively seize the multifaceted XRD sample evaluation in a two-dimensional aircraft.”
Utilizing a neural network, the researchers utilized the auto-encoder to 150 XRD patterns of magnetic alloys with totally different concentrations. Within the function area, every XRD is projected to a single point. These factors type clusters, by which comparable supplies with comparable constituent concentrations are positioned nearer collectively. Thus, the space between the factors within the function area permits the estimation of the focus of any given pattern alloy. This additionally permits the fine-tuning of alloys by not directly figuring out the XRD peaks that change when new components are added to an alloy or its constituent factor ratios are altered.
The researchers additional proposed a novel software of the function area. When a peak of curiosity is masked on the unique XRD sample, the purpose on the function area shifts. The extent of the shift helps distinguish how related a peak is to capturing the properties of a cloth. Utilizing this method, the researchers have been in a position to determine which peak is definitely related to be watched out for estimating the quantity of doping and many others.—one thing that would not have been predicted by a human however was revealed utilizing Deep Studying.
The researchers additionally proposed the appliance of the auto-encoder for the technology of synthetic XRD patterns by interpolating present ones to deal with tiny modifications in alloy compositions. The strategy would generate believable datasets, avoiding computationally costly ab initio simulations.
“The outcomes of this analysis aren’t restricted to XRD peak patterns. Somewhat, they supply a common Deep Studying method that can be utilized to extract options from materials science knowledge. Its framework can discover hidden regularity in nature that isn’t identifiable by people and is anticipated to function a strong instrument for theorem discovery via knowledge science,” says Prof. Maezono.
The appliance of the described auto-encoder may speed up the event of excessive effectivity, low value, and low environmental influence supplies, ushering in a brand new period of Deep Studying-based supplies science analysis.
Extra info:
Keishu Utimula et al, Characteristic House of XRD Patterns Constructed by an Autoencoder, Superior Concept and Simulations (2022). DOI: 10.1002/adts.202200613
Supplied by
Japan Superior Institute of Science and Expertise
Quotation:
Discovering hidden regularities in nature: Researchers apply deep studying to X-ray diffraction (2022, December 22)
retrieved 22 December 2022
from https://phys.org/information/2022-12-hidden-regularities-nature-deep-x-ray.html
This doc is topic to copyright. Aside from any honest dealing for the aim of personal research or analysis, no
half could also be reproduced with out the written permission. The content material is offered for info functions solely.